Results
User Study
The following figure shows the results of a userstudy. We can see that our method is prefered over BPG, even when BPG uses almost twice the number of bits.
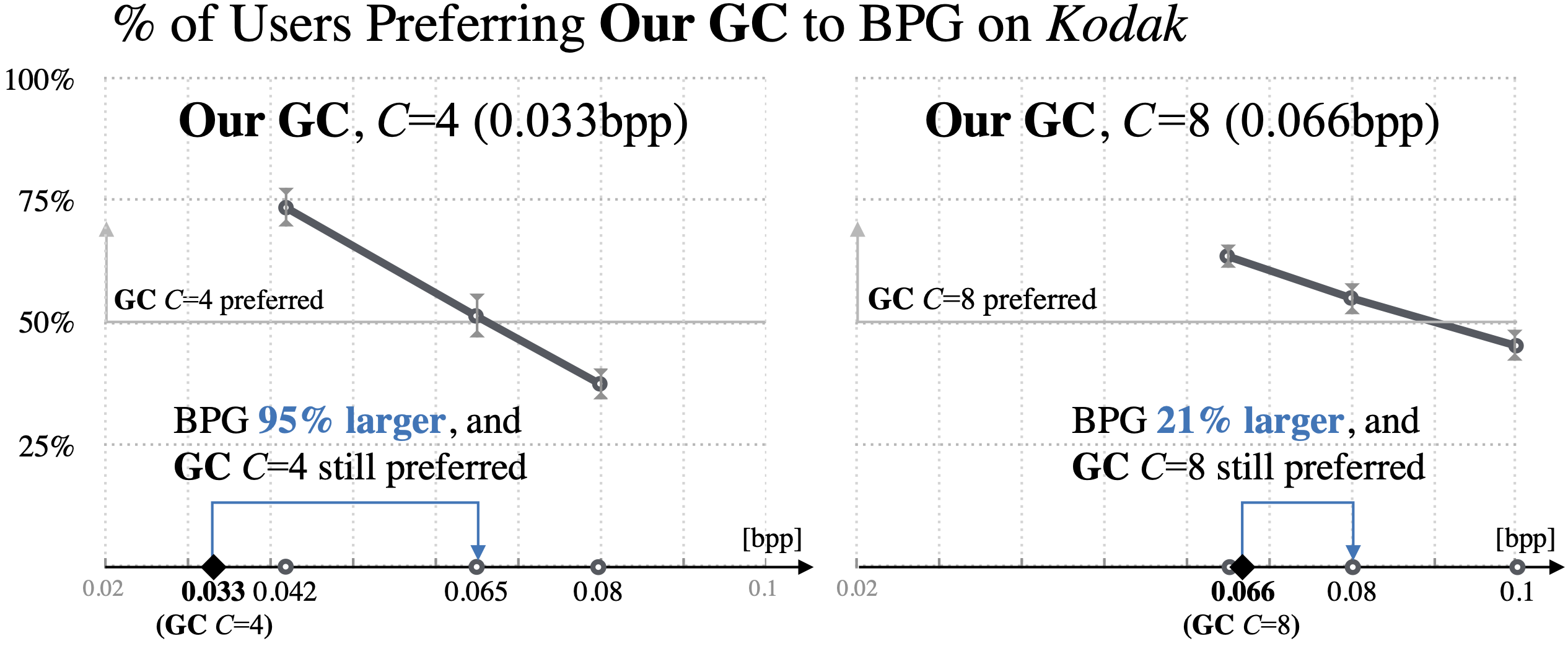
The bitrate of our models is highlighted on the x-axis with
a black diamond. The thick gray line shows the percentage of users
preferring our model to BPG at that bitrate (bpp). The blue arrow
points from our model to the highest-bitrate BPG operating point
where more than 50% of users prefer ours, visualizing how many
more bits BPG uses at that point.
In the paper, we obtain similar results for the RAISE1k dataset and Cityscapes.
Semantic Preservation
We measure the preservation of semantics through the mIoU of a pre-trained PSPNet for semantic segmentation on CityScapes in the following Figure.
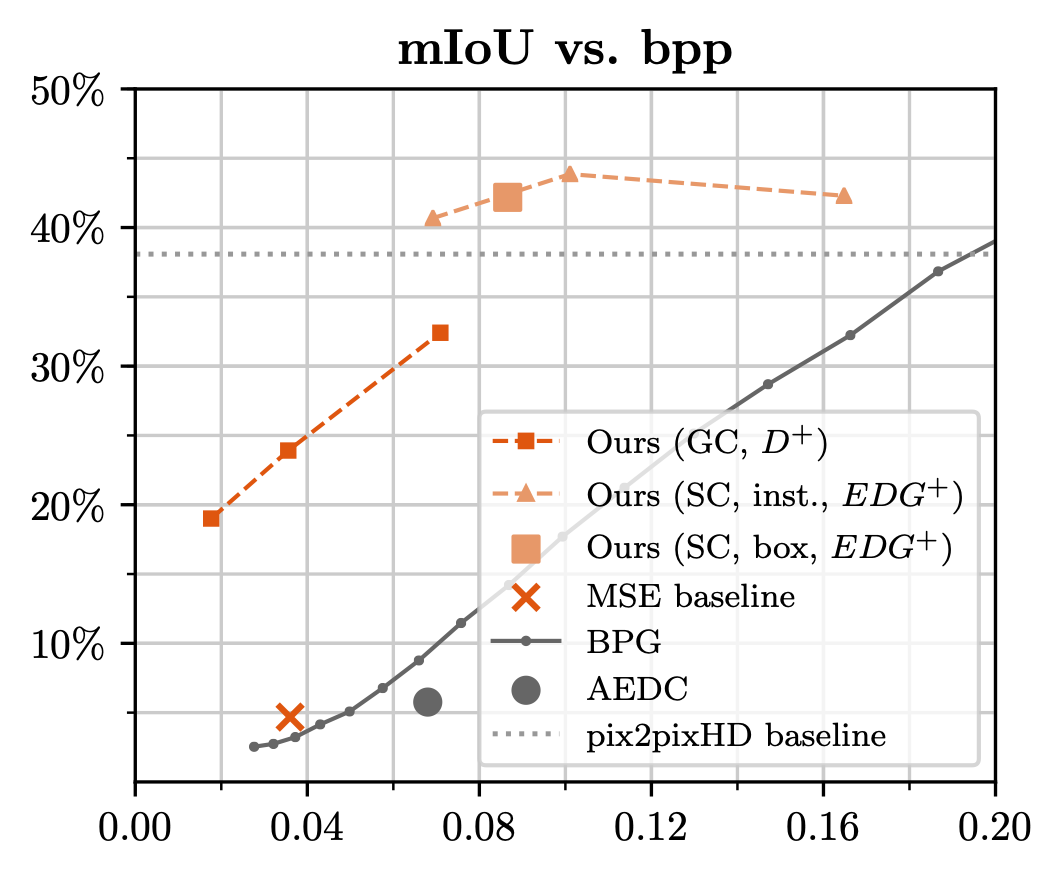
We obtain a significantly higher mIoU compared to BPG, which is further improved when guiding the training with semantics.
Selective Synthesis
Our method allows for selectively preserving some regions while fully synthesizing the rest of the image (keeping the semantics in tact).
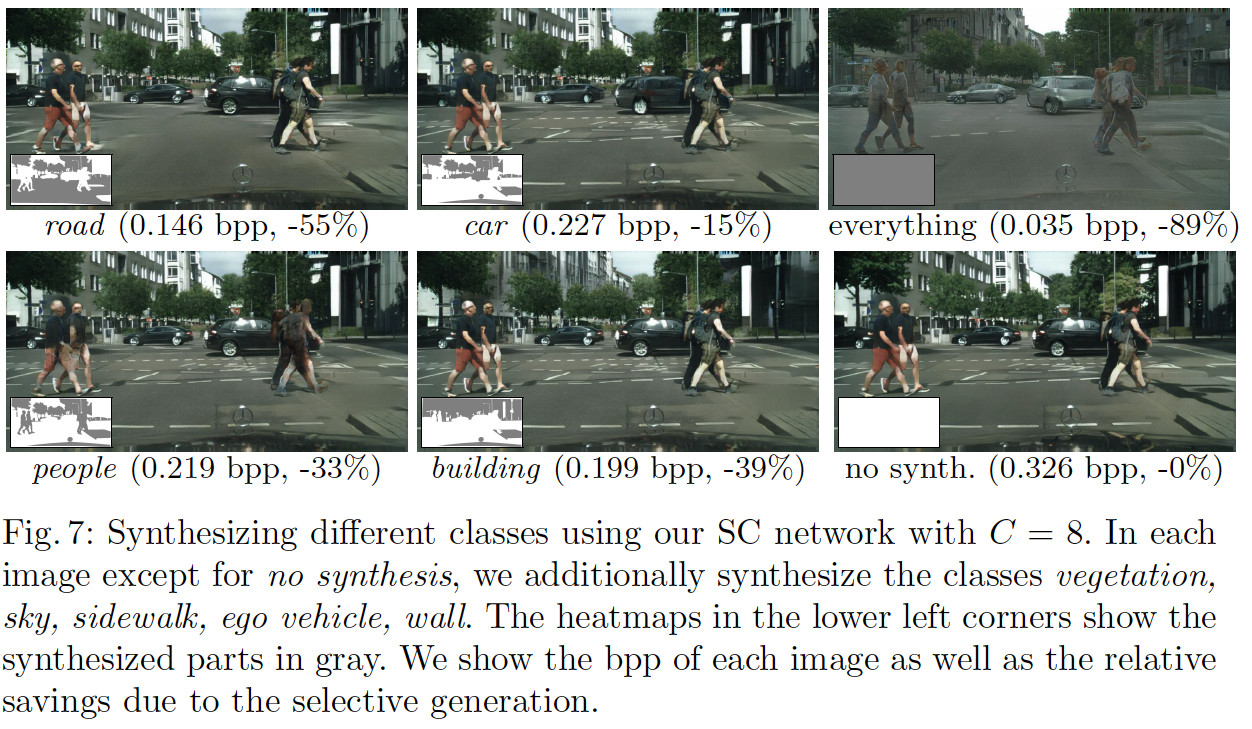
Check the the paper for more details!